Learning in the visual cortex
Hebbian learning refers to the fundamental principle of learning in brain. We investigate the precise implementation required to learn useful information representations and prosessings similar to those of the primate brain. We focus on how appropriate visual representations develop and how the highly recurrent connectivity structure of the neocortex affects them.
Biologically grounded deep neural networks of the visual cortex

The requirements on a biologically grounded neural network model of the visual cortex are many. First of all it has to provide realistic processing, which comprises that neurons have biologically relaistic receptive fields, connectivity, and dynamics. Our approach is to utilize Hebbian learning mechanisms within complex recurrent neural systems for their self-organization. Therefore, basic learning principles have to be advanced by, for instance, homeostatic mechanisms for stabilization, temporal traces, intrinsic as well as structural plasticity mechanisms to achieve realistic and unbiased results.
One essential capability of the brain is to form invariant representation against the permanently ongoing changes of our environment. In the visual system this is the invariance to object transformations. We investigted Hebbian learning advanced with post-synaptic calcium traces as a natural implementation of the so called slowness principle for learning invariant visual representations on natural scenes (Teichmann et al. 2012).
To allow for informative representations in deeper neural networks homeostatic setpoints are key for the neural system. We found in intrinsic plasticity a critical mechanism, controlling the operating point of the neurons to have equal mean and variance (Teichmann et al. 2015). By its reduction of the differences in the neural activity distributions it provides a balanced representation of information, so that deeper layers carry more informative neural codes than without having an similar operating point.
Realistic models of the visual cortex are very complex in terms of their connectome. The lack of precise data down to the level of individual conncetions, as well as the bias the modeller decisions have on the learnings of the system, impact the value of the results of a computational model. Moreover, the brain is not static and underlies ongoing changes even in adulthood. We developed a structural plasticity mechanism which continuously modifies the network connectivity, so-called experience-dependent spatial growth (Teichmann 2018). It removes initial restrictions from the neurons connectivity and allows them to develop more realistic receptive fields.
With that at hand networks develop V1 and V2 receptive fields with comparable properties in their natural texture sensitivity to macaque monkey data (Teichmann 2018). Also their connection statistics have been comparable to animal recordings, where synapses between neurons with high response correlations also form strong synapses and these few synapses contribute the majority of the input to the neurons. These neural networks with their high degree of biologically plausibility are evaluated on standard object recognition benchmarks and could achieve good results (Teichmann et al. 2021).
Rate-based neural network
|
Spike-based neural network
|
---|
Table 1: Accuracy values for different layers and neuron types of a rate-based and spiking-based neural network on the MNIST dataset. Data from (Teichmann et al. 2021).
The role of inhibitory plasticity for robust and efficient representations
Biologically grounded neural networks rely on a multitude of mechanisms to form their representations. One key mechanism is inhibition, which shapes the neural codes towards towards efficient and robust representations. We found that neural codes are more robust to noise occlusions when a competitive mechanism is present, such as recurrent lateral inhibition (Kermani et al. 2015, Larisch et al. 2018). We could also show that inhibition has to be plastic during development to achieve high representational efficiency of neural codes (Larisch et al. 2021).
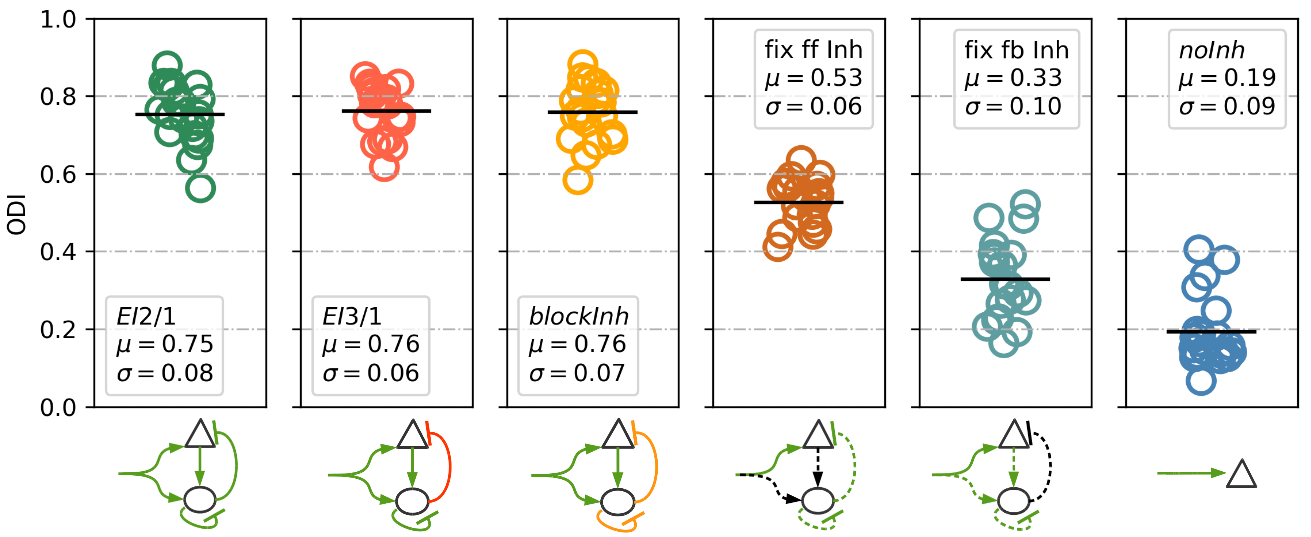
The role of recurrence for robust task relevant representations, attention, and guidance (dynamic control)
It is still an open question how the cortex develops highly behavior relevant representations and dynamically utilizes them. One key mechanism is its high degree of recurrence, which includes the influence of deeply processed information on upstream areas. Cortical feedback is known as neural correlate of attention, which can modulate neuron activities. However, it is little known how such processes can guide cortical plasticity. We investigate important circuit motives which deliver feedback (e.g. Beuth et al. 2015), how this feedback interferes with Hebbian synaptic plasticity and facilates phenomenons such as visual attention. This comprises the role of multiple systems which interact with the visual system and guide it to behavior relevant representations, such as the amygdala, contributing the biological valence of objects (Liebold et al. 2015), or the basal-ganglia for category learning (Villagrasa et al. 2018).
Since detailed biologically grounded neural networks provide a multitude of mechanism mutually influencing the processing the role of the single mechanisms beyond rapid object recognition is questioned. We systematically vary network properties to assess their contribution, for example, to the robustness of the human brain to visual perturbations. One important aspect of deep recurrent systems is their stability against runaway dynamics. To achieve highly recurrent deep and stable neural networks we combine homeostatic setpoints in excitatory and inhibitory plasticity, as well as adaptation mechanisms of neurons.
Associated projects
ESF and SAB Landesinnovationspromotionstipendium "DeepLearning- Robuste Objekterkennung für intelligente Roboter". (2017-2020)
Graduiertenkolleg Crossworlds - Kopplung virtueller und realer sozialer Welten. DFG GRK 1780/1 (2012-2018)
BMBF program "US-German collaboration on computational neuroscience": Model-driven single-neuron studies of cortical remapping in the dorsal and ventral visual streams. BMBF 01GQ1409 (2015-2018).
DFG grant "Computational modeling of reentrant processing for visual masking and attention". DFG HA 2630/6-1 (2010-2013).
Selected Publications
Larisch, R., Gönner, L., Teichmann, M., Hamker, F. H. (2021).
Sensory coding and contrast invariance emerge from the control of plastic inhibition over emergent selectivity.
PLOS Computational Biology, 17, (1-37), doi:10.1371/journal.pcbi.1009566
Teichmann, M., Larisch, R., Hamker, F. H. (2021).
Performance of biologically grounded models of the early visual system on standard object recognition tasks.
Neural Networks, 144, (210-228). doi:10.1016/j.neunet.2021.08.009
Teichmann, M. (2018).
A plastic multilayer network of the early visual system inspired by the neocortical circuit.
Dissertation, Technische Universität Chemnitz, http://nbn-resolving.de/urn:nbn:de:bsz:ch1-qucosa2-318327
Larisch, R., Teichmann, M., & Hamker, F. H. (2018).
A neural spiking approach compared to deep feedforward networks on stepwise pixel erasement.
In V. Kůrková, Y. Manolopoulos, B. Hammer, L. Iliadis, & I. Maglogiannis (Eds.), Artificial neural networks and machine learning – icann 2018 (pp. 253–262) Cham: Springer International Publishing. doi:10.1007/978-3-030-01418-6_25
Teichmann, M., & Hamker, F. (2015).
Intrinsic plasticity: A simple mechanism to stabilize hebbian learning in multilayer neural networks.
In T. Villmann & F.-M. Schleif (Eds.), Proc Workshop new challenges in neural computation - nc2 2015, machine learning reports (p. 103-111)
Kermani Kolankeh, A., Teichmann, M., & Hamker, F. H. (2015).
Competition improves robustness against loss of information.
Frontiers in Computational Neuroscience, 9 , 35.
doi:10.3389/fncom.2015.00035
Liebold, B., Richter, R., Teichmann, M., Hamker, F. H., & Ohler, P. (2015).
Human capacities for emotion recognition and their implications for computer vision.
i-com, 14 (2), 126–137. doi:10.1515/icom-2015-0032
Teichmann, M., Wiltschut, J., & Hamker, F. (2012).
Learning invariance from natural images inspired by observations in the primary visual cortex.
Neural Computation, 24 (5), 1271-1296. doi:10.1162/NECO_a_00268
Wiltschut, J., & Hamker, F. H. (2009).
Efficient coding correlates with spatial frequency tuning in a model of V1 receptive field organization.
Visual Neuroscience, 26(1), 21-34. doi:10.1017/S0952523808080966